|
|
Line 13: |
Line 13: |
| <div>Adversarial Deepfakes: Recent advances in video manipulation techniques have made the generation of fake videos more accessible than ever before. Manipulated videos can fuel disinformation and reduce trust in the media. Therefore detection of fake videos has garnered immense interest in academia and industry. Recently developed Deepfake detection methods rely on deep neural networks (DNNs) to distinguish AI-generated fake videos from real videos. In this work, we demonstrate that it is possible to bypass such detectors by adversarially modifying fake videos synthesized using existing Deepfake generation methods. We further demonstrate that our adversarial perturbations are robust to image and video compression codecs, making them a real-world threat. We present pipelines in both white-box and black-box attack scenarios that can fool DNN based Deepfake detectors into classifying fake videos as real.<ref>Shehzeen: please add a paragraph about adversarial attacks on Deepfakes and add a picture</ref></div> | | <div>Adversarial Deepfakes: Recent advances in video manipulation techniques have made the generation of fake videos more accessible than ever before. Manipulated videos can fuel disinformation and reduce trust in the media. Therefore detection of fake videos has garnered immense interest in academia and industry. Recently developed Deepfake detection methods rely on deep neural networks (DNNs) to distinguish AI-generated fake videos from real videos. In this work, we demonstrate that it is possible to bypass such detectors by adversarially modifying fake videos synthesized using existing Deepfake generation methods. We further demonstrate that our adversarial perturbations are robust to image and video compression codecs, making them a real-world threat. We present pipelines in both white-box and black-box attack scenarios that can fool DNN based Deepfake detectors into classifying fake videos as real.<ref>Shehzeen: please add a paragraph about adversarial attacks on Deepfakes and add a picture</ref></div> |
|
| |
|
| <div></div> | | <div><!-- You can add a image here --></div> |
| <div>Placeholder.<ref>Shehzeen: please add a paragraph about adversarial attacks and defense on Speech Recognition Models and add a picture</ref></div> | | <div>Placeholder.<ref>Shehzeen: please add a paragraph about adversarial attacks and defense on Speech Recognition Models and add a picture</ref></div> |
|
| |
|
Line 28: |
Line 28: |
| As data-mining algorithms are being incorporated into today’s technology, concerns about data privacy are rising. The ACES Lab has been contributing to the field of privacy-preserving computing for more than a decade now, and it still remains one of the pioneers in the area. Several signature research domains of our group are listed below. | | As data-mining algorithms are being incorporated into today’s technology, concerns about data privacy are rising. The ACES Lab has been contributing to the field of privacy-preserving computing for more than a decade now, and it still remains one of the pioneers in the area. Several signature research domains of our group are listed below. |
| <div class="research-grid"> | | <div class="research-grid"> |
| <div></div> | | <div><!-- You can add a image here --></div> |
| <div>Our group is dedicated to execution of Secure Function Evaluation (SFE) protocols in practical time limits. Along with algorithmic optimizations, we are working on acceleration of the inherent computation by designing application specific accelerators. [https://github.com/ACESLabUCSD/FASE FASE] [FCCM'19], currently the fastest accelerator for the Yao's Garbled Circuit (GC) protocol is designed by the ACES Lab. It outperformed the previous works by a minimum of 110 times in terms of throughput per core. Our group also developed [https://dl.acm.org/doi/10.1145/3195970.3196074 MAXelerator] [DAC'18] - an FPGA accelerator for GC customized for matrix multiplication. It is 4 times faster than FASE for this particular operation. MAXelerator is one of the examples of co-optimizations of the algorithm and the underlying hardware platform. Our current projects include acceleration of Oblivious Transfer (OT) and Homomorphic Encryption (HE). Moreover, as part of our current efforts to develop practical privacy-preserving Federated Learning (FL), we are working on evaluation of the existing hardware cryptographic primitives on Intel processors and devising new hardware-based primitives that complement the available resources. Our group plans to design efficient systems through the co-optimization of the FL algorithms, defense mechanisms, cryptographic primitives, and the hardware primitives. <ref>Siam: please add tinygarble and add a picture for all of the papers mentioned in this paragraph.</ref></div> | | <div>Our group is dedicated to execution of Secure Function Evaluation (SFE) protocols in practical time limits. Along with algorithmic optimizations, we are working on acceleration of the inherent computation by designing application specific accelerators. [https://github.com/ACESLabUCSD/FASE FASE] [FCCM'19], currently the fastest accelerator for the Yao's Garbled Circuit (GC) protocol is designed by the ACES Lab. It outperformed the previous works by a minimum of 110 times in terms of throughput per core. Our group also developed [https://dl.acm.org/doi/10.1145/3195970.3196074 MAXelerator] [DAC'18] - an FPGA accelerator for GC customized for matrix multiplication. It is 4 times faster than FASE for this particular operation. MAXelerator is one of the examples of co-optimizations of the algorithm and the underlying hardware platform. Our current projects include acceleration of Oblivious Transfer (OT) and Homomorphic Encryption (HE). Moreover, as part of our current efforts to develop practical privacy-preserving Federated Learning (FL), we are working on evaluation of the existing hardware cryptographic primitives on Intel processors and devising new hardware-based primitives that complement the available resources. Our group plans to design efficient systems through the co-optimization of the FL algorithms, defense mechanisms, cryptographic primitives, and the hardware primitives. <ref>Siam: please add tinygarble and add a picture for all of the papers mentioned in this paragraph.</ref></div> |
|
| |
|
Line 50: |
Line 50: |
| <div>The success of contemporary Machine Learning (ML) methods relies on careful design parameter choices, often made by (human) domain experts. With the ever growing range of smart applications and the continuous increase in the complexity of state-of-the-art models, automated ML design (AutoML) methodologies are gaining traction. We target AutoML through the lens of multi-objective optimization and propose a methodology, dubbed [https://ieeexplore.ieee.org/document/9086078 AdaNS], based on Adaptive Non-uniform Sampling that performs simultaneous optimization of conflicting objectives inherent in ML system design. AdaNs is applicable in a variety of applications where the goal is to perform automated parameter selection for system design under a set of constraints and objectives. A prominent property of AdaNS is its high scalability which supports parameter spaces larger than 10130. So far we have applied AdaNS in the context of AutoML for hardware-aware Deep Neural Network (DNN) compression where a state-of-the-art compute-intensive DNN is cusotmized for enhanced performance on a target hardware platform while preserving its accuracy.</div> | | <div>The success of contemporary Machine Learning (ML) methods relies on careful design parameter choices, often made by (human) domain experts. With the ever growing range of smart applications and the continuous increase in the complexity of state-of-the-art models, automated ML design (AutoML) methodologies are gaining traction. We target AutoML through the lens of multi-objective optimization and propose a methodology, dubbed [https://ieeexplore.ieee.org/document/9086078 AdaNS], based on Adaptive Non-uniform Sampling that performs simultaneous optimization of conflicting objectives inherent in ML system design. AdaNs is applicable in a variety of applications where the goal is to perform automated parameter selection for system design under a set of constraints and objectives. A prominent property of AdaNS is its high scalability which supports parameter spaces larger than 10130. So far we have applied AdaNS in the context of AutoML for hardware-aware Deep Neural Network (DNN) compression where a state-of-the-art compute-intensive DNN is cusotmized for enhanced performance on a target hardware platform while preserving its accuracy.</div> |
|
| |
|
| <div></div> | | <div><!-- You can add a image here --></div> |
| <div>Placeholder.<ref>Shehzeen: please add a paragraph+picture for Hardware Acceleration of DNN based Speech Synthesis Model</ref></div> | | <div>Placeholder.<ref>Shehzeen: please add a paragraph+picture for Hardware Acceleration of DNN based Speech Synthesis Model</ref></div> |
|
| |
|
The ACES lab is conducting interdisciplinary research in robust ML, privacy-preserving technologies, automation and hardware, IP protection, and emerging technologies.
Machine Learning (ML) models are often trained to satisfy a certain measure of performance such as classification accuracy, object detection accuracy, etc. In safety-sensitive tasks, a reliable ML model should satisfy reliability and robustness tests in addition to accuracy. Our group has made several key contributions to the development of robustness tests and safeguarding methodologies to enhance the reliability of ML systems. Some example projects are listed below:
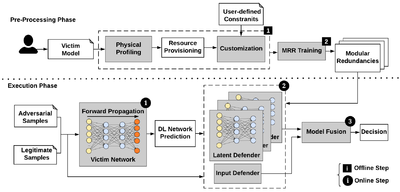
Recent advances in adversarial Deep Learning (DL) have opened up a largely unexplored surface for malicious attacks jeopardizing the integrity of autonomous DL systems. With the wide-spread usage of DL in critical and time-sensitive applications, including unmanned vehicles, drones, and video surveillance systems, online detection of malicious inputs is of utmost importance. In a project called
DeepFense, we propose the first end-to-end automated framework that simultaneously enables efficient and safe execution of DL models. DeepFense formalizes the goal of thwarting adversarial attacks as an optimization problem that minimizes the rarely observed regions in the latent feature space spanned by a DL network. To solve the aforementioned minimization problem, a set of complementary but disjoint modular redundancies are trained to validate the legitimacy of the input samples in parallel with the victim DL model. DeepFense leverages hardware/software/algorithm co-design and customized acceleration to achieve just-in-time performance in resource-constrained settings. The proposed countermeasure is unsupervised, meaning that no adversarial sample is leveraged to train modular redundancies. We further provide an accompanying API to reduce the non-recurring engineering cost and ensure automated adaptation to various platforms. Extensive evaluations on FPGAs and GPUs demonstrate up to two orders of magnitude performance improvement while enabling online adversarial sample detection.
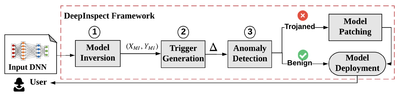
Large-scale DL models are typically developed and trained by third-party companies that have sufficient computation power. These pre-trained models are then delivered to end users for real-world deployment. Such a supply chain raises security concerns about the pre-trained models obtained from untrusted parties. In our work DeepInspect, we propose the first black-box neural Trojan detection and mitigation framework that examines whether a given trained DL model has been backdoored during its training pipeline. DeepInspect leverages conditional GAN to emulate the backdoor attack and recovers potential Trojan triggers. We then use the footprint of the reconstructed triggers as the test metrics of hypothesis testing to determine the probability of Trojan insertion. In addition to reliable model-level Trojan detection, DeepInspect further enables the end users to improve model robustness by patching the pre-trained model with the perturbed inputs from the conditional generator with correct labels.
[1]
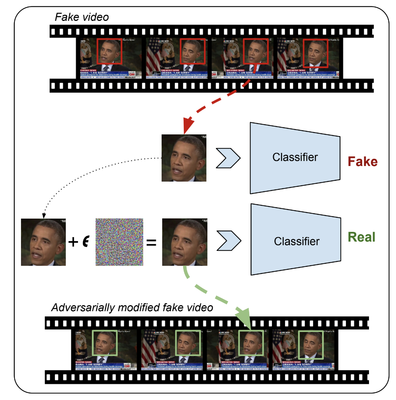
Adversarial Deepfakes: Recent advances in video manipulation techniques have made the generation of fake videos more accessible than ever before. Manipulated videos can fuel disinformation and reduce trust in the media. Therefore detection of fake videos has garnered immense interest in academia and industry. Recently developed Deepfake detection methods rely on deep neural networks (DNNs) to distinguish AI-generated fake videos from real videos. In this work, we demonstrate that it is possible to bypass such detectors by adversarially modifying fake videos synthesized using existing Deepfake generation methods. We further demonstrate that our adversarial perturbations are robust to image and video compression codecs, making them a real-world threat. We present pipelines in both white-box and black-box attack scenarios that can fool DNN based Deepfake detectors into classifying fake videos as real.
[2]
We also explore security threats and defense frameworks for Machine Learning models employed in audio/speech processing domains
Universal Adversarial Perturbations for Speech Recognition Systems: In this work, we demonstrate the existence of universal adversarial audio perturbations that cause mis-transcription of audio signals by automatic speech recognition (ASR) systems. We propose an algorithm to find a single quasi-imperceptible perturbation, which when added to any arbitrary speech signal, will most likely fool the victim speech recognition model. Our experiments demonstrate the application of our proposed technique by crafting audio-agnostic universal perturbations for the state-of-the-art ASR system – Mozilla DeepSpeech. Additionally, we show that such perturbations generalize to a significant extent across models that are not available during training, by performing a transferability test on a WaveNet based ASR system.
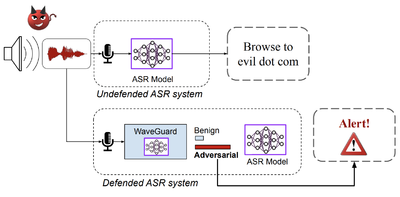
Waveguard Defense Framework for Speech Recognition Systems: There has been a recent surge in adversarial attacks on deep learning based automatic speech recognition (ASR) systems. These attacks pose new challenges to deep learning security and have raised significant concerns in deploying ASR systems in safety-critical applications. In this work, we introduce WaveGuard: a framework for detecting adversarial inputs that are crafted to attack ASR systems. Our framework incorporates audio transformation functions and analyses the ASR transcriptions of the original and transformed audio to detect adversarial inputs. We demonstrate that our defense framework is able to reliably detect adversarial examples constructed by four recent audio adversarial attacks, with a variety of audio transformation functions. With careful regard for best practices in defense evaluations, we analyze our proposed defense and its strength to withstand adaptive and robust attacks in the audio domain. We empirically demonstrate that audio transformations that recover audio from perceptually informed representations can lead to a strong defense that is robust against an adaptive adversary even in a complete white-box setting. Furthermore, WaveGuard can be used out-of-the box and integrated directly with any ASR model to efficiently detect audio adversarial examples, without the need for model retraining.
Privacy-preserving technologies[edit | edit source]
As data-mining algorithms are being incorporated into today’s technology, concerns about data privacy are rising. The ACES Lab has been contributing to the field of privacy-preserving computing for more than a decade now, and it still remains one of the pioneers in the area. Several signature research domains of our group are listed below.
Our group is dedicated to execution of Secure Function Evaluation (SFE) protocols in practical time limits. Along with algorithmic optimizations, we are working on acceleration of the inherent computation by designing application specific accelerators.
FASE [FCCM'19], currently the fastest accelerator for the Yao's Garbled Circuit (GC) protocol is designed by the ACES Lab. It outperformed the previous works by a minimum of 110 times in terms of throughput per core. Our group also developed
MAXelerator [DAC'18] - an FPGA accelerator for GC customized for matrix multiplication. It is 4 times faster than FASE for this particular operation. MAXelerator is one of the examples of co-optimizations of the algorithm and the underlying hardware platform. Our current projects include acceleration of Oblivious Transfer (OT) and Homomorphic Encryption (HE). Moreover, as part of our current efforts to develop practical privacy-preserving Federated Learning (FL), we are working on evaluation of the existing hardware cryptographic primitives on Intel processors and devising new hardware-based primitives that complement the available resources. Our group plans to design efficient systems through the co-optimization of the FL algorithms, defense mechanisms, cryptographic primitives, and the hardware primitives.
[4]
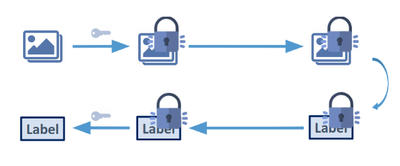
Advancements in deep neural networks have fueled machine learning as a service, where clients receive an inference service from a cloud server. Many applications such as medical diagnosis and financial data analysis require the client’s data to remain private. The objective of privacy-preserving inference is to use secure computation protocols to perform inference on the client’s data, without revealing the data to the server. Towards this goal, the ACES lab has been conducting exemplary research in the intersection DNN inference and secure computation. We have developed several projects including
DeepSecure,
Chameleon,
XONN,
SlimBin, COINN, and several on-going projects. The core idea of our work is to co-optimize machine learning algorithms with secure execution protocols to achieve a better tradeoff between accuracy and runtime. Using this co-optimization approach, our customized DNN inference solutions have achieved significant runtime improvements over contemporary work.
Many data-mining algorithms and machine learning techniques were originally developed to run on high-end GPU clusters that have massive computing capacity and power consumption. Many real-world applications require these algorithms to run on embedded devices where the computing capacity and power budget are limited. The ACES group has developed several novel innovations in the areas of DNN compression/customization, hardware design for ML, and design automation for efficient machine learning. Several example projects are listed below:
LookNN is a deep learning acceleration tool that removes all multiplications from the computational flow of neural networks and replaces them with look-up table search. First, we develop a pre-processing algorithm along with theoretical analysis to convert the multiplications into lookup tables without loss of classification accuracy in the neural networks. Second, thanks to advances in
memory-based computing, we utilize Ternary Content Addressable Memory (TCAM) architectures to implement efficient lookup table search. We incorporated LookNN engines into an AMD GPU and simulated the performance gain, showing an average of 2.2× energy reduction and 2.5× runtime improvement.
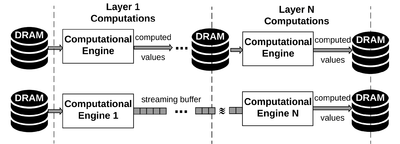
Encodeep is an end-to-end framework that facilitates encoding, bitwidth customization, fine-tuning, and implementation of neural networks on FPGA platforms. EncoDeep incorporates nonlinear encoding to the computation flow of neural networks to save memory. The encoded features demand significantly lower storage compared to the raw full-precision activation values; therefore, the execution flow of EncoDeep hardware engine is completely performed within the FPGA using on-chip streaming buffers with no access to the off-chip DRAM. We further propose a fully automated optimization algorithm that determines the flexible encoding bitwidths across network layers. EncoDeep full-stack framework comprises a compiler that takes a high-level Python description of an arbitrary neural network. The compiler then instantiates the corresponding elements from EncoDeep Hardware library for FPGA implementation. Our evaluations on MNIST, SVHN, and CIFAR-10 datasets demonstrate an average of 4.65× throughput improvement compared to stand-alone weight encoding. We further compare EncoDeep with six FPGA accelerators on ImageNet, showing an average of 3.6× and 2.54× improvement in throughput and performance-per-watt, respectively.
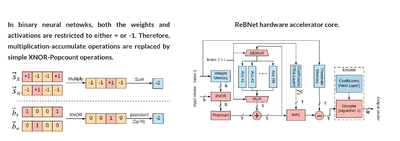
Binary neural networks are excellent choices for low-power execution of deep learning. However, the accuracy of binary networks is usually low. To solve this problem,
ReBNet extends them to support multi-level (residual) binarization: a scheme that improves the classification accuracy but still enjoys the hardware simplicity of binary neural networks. This project won the best paper award in FCCM 2018.
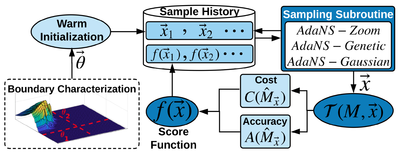
The success of contemporary Machine Learning (ML) methods relies on careful design parameter choices, often made by (human) domain experts. With the ever growing range of smart applications and the continuous increase in the complexity of state-of-the-art models, automated ML design (AutoML) methodologies are gaining traction. We target AutoML through the lens of multi-objective optimization and propose a methodology, dubbed
AdaNS, based on Adaptive Non-uniform Sampling that performs simultaneous optimization of conflicting objectives inherent in ML system design. AdaNs is applicable in a variety of applications where the goal is to perform automated parameter selection for system design under a set of constraints and objectives. A prominent property of AdaNS is its high scalability which supports parameter spaces larger than 10130. So far we have applied AdaNS in the context of AutoML for hardware-aware Deep Neural Network (DNN) compression where a state-of-the-art compute-intensive DNN is cusotmized for enhanced performance on a target hardware platform while preserving its accuracy.
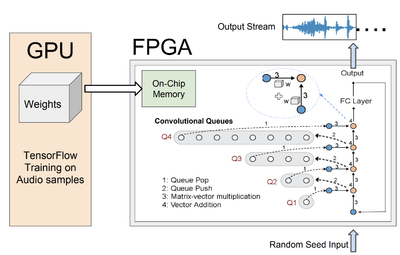
Autoregressive convolutional neural networks (CNNs) have been widely exploited for sequence generation tasks such as audio synthesis, language modeling and neural machine translation. WaveNet is a deep autoregressive CNN composed of several stacked layers of dilated convolution that is used for sequence generation. While WaveNet produces state-of-the art audio generation results, the naive inference implementation is quite slow; it takes a few minutes to generate just one second of audio on a high-end GPU. We develop the first accelerator platform FastWave for autoregressive convolutional neural networks, and address the associated design challenges. We design the Fast-Wavenet inference model in Vivado HLS and perform a wide range of optimizations including fixed-point implementation, array partitioning and pipelining. Our model uses a fully parameterized parallel architecture for fast matrix-vector multiplication that enables per-layer customized latency fine-tuning for further throughput improvement. Our experiments comparatively assess the tradeoff between throughput and resource utilization for various optimizations. Our best WaveNet design on the Xilinx XCVU13P FPGA that uses only on-chip memory, achieves 66× faster generation speed compared to CPU implementation and 11× faster generation speed than GPU implementation.
Designing and training DNNs with high-performance is both time- and resource consuming. As such, pre-trained DNNs shall be considered as the intellectual property (IP) of the model developer and need to be protected against copyright infringement attacks. Several projects of this category are listed below:[6]
We propose the first dynamic DNN watermarking framework (
DeepSigns) to tackle the IP protection problem. DeepSigns embeds the signature of the model owner into the activation maps of the marked model before model distribution. In Particular, we design a watermark (WM)-specific regularization loss and embed the WM via a few rounds of model re-training. The inserted signature can be later verified for ownership proof. DeepSigns is applicable in both white-box and black-box model deployment scenarios.
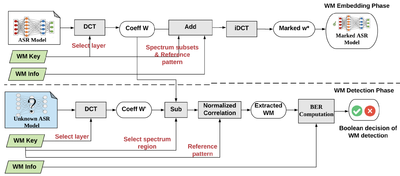
As an orthogonal approach to spatial watermarking technique DeepSigns, we present the first spectral DNN watermarking framework. SpecMark identifies the significant frequency components of the model parameters and encodes the owner’s WM in the corresponding spectrum region before sharing the model with end-users. The model builder can later extract the spectral WM to verify his ownership of the marked model. We demonstrate the effectiveness of SpecMark on DNN-based automated speech recognition (ASR) systems.
We propose
DeepMarks, the first DNN fingerprinting framework that is able to provide both ownership verification and unique user identification. DeepMarks embeds a unique fingerprint for each user in the model parameter distribution. The embedded fingerprint can be extracted for model IP protection and Digital Right Management (DRM).
As an extension, we develop
DeepAttest, the first on-device DNN attestation framework that provides IP protection at the hardware level. DeepAttest works by designing a device-specific fingerprint which is encoded in the weights of the DNN deployed on the target platform. The embedded fingerprint (FP) is later extracted with the support of the Trusted Execution Environment (TEE). The existence of the pre-defined FP is used as the attestation criterion to determine whether the queried DNN is authenticated. Our attestation framework ensures that only authorized DNN programs yield the matching FP and are allowed for inference on the target device.
WIP
Zero knowledge proofs (Nojan)[edit | edit source]
WIP
Machine Learning for media (Shehzeen)[edit | edit source]
WIP
- ↑ huili please double check the text and add a picture
- ↑ Shehzeen: please add a paragraph about adversarial attacks on Deepfakes and add a picture
- ↑ Shehzeen: please add a paragraph about adversarial attacks and defense on Speech Recognition Models and add a picture
- ↑ Siam: please add tinygarble and add a picture for all of the papers mentioned in this paragraph.
- ↑ Shehzeen: please add a paragraph+picture for Hardware Acceleration of DNN based Speech Synthesis Model
- ↑ Huili: please expand each paper, and put a picture for each of them.